Quantifying the Amount of Uncertainty in Single Studies Caused by Measurement Error
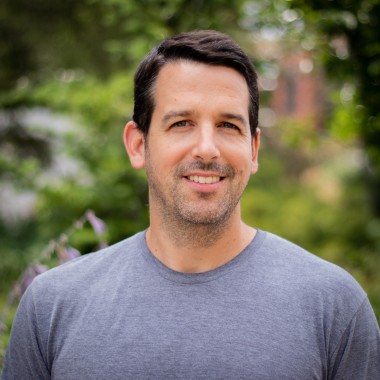
About the Project
Interpreting single studies and trying to apply their findings to solve practical problems can be challenging. This is because specific findings are rarely definitive due to the many sources of error that can mask the true effect under investigation. To combat this, researchers are frequently trained to recognize and mitigate as many sources of errors possible. Of late, researchers and consumers of research have become increasingly aware of additional, more intentional, sources of error including questionable research practices and data fraud. This awareness, paired with widely publicized replication failures, has caused expectations regarding the quality and utility of research to adjust. Importantly, these events have highlighted the importance of being aware of all the sources of error that can alter research findings. Understanding which errors affect data can help to ensure that scientific results are properly interpreted and applied. Our program of research is aimed at identifying and quantifying the degree of uncertainty introduced by an additional, often over-looked, source of error: measurement error.
Measurement error is present in every study, yet its potential to alter a single study's results is not currently well understood. Our previous research has illustrated that even modest amounts of measurement error can make the results from two perfectly executed studies look drastically different from one another. This finding can come as a surprise to those who have been trained under Classical Test Theory (CTT). CTT can give the impression that measurement error always has an attenuating effect on observed relations. However, the expectation that measurement error has a consistent attenuating effect is the result of an oversimplification of CTT. Specifically, it is the result of applying a population-level theory to the sample-level without recognizing important differences between samples and populations. Namely, CTT has important assumptions that hold true at the population-level, but these assumptions are violated at the sample-level. These assumption violations cause measurement error to have a random effect on data at the sample-level. Our research extends these ideas and is designed to quantify the degree of uncertainty in a result that is due to measurement error. To do so, we will create an interval-based statistic that will indicate the range of results that can be expected in a replication due to measurement error.
The development of our statistic will unfold in two phases and will occur through the use of computer simulations. Phase 1, will generate equations to estimate the expected variability in results with two large scale computer simulations. We will derive estimates of the expected variability of research results by manipulating a number of study-level parameters to test how the accuracy of a result changes as these parameters change. The results of these simulations will then be used to generate arithmetical equations to calculate the upper and lower bounds of our interval. Computer simulations are ideal for this application as simulations make it possible to repeatedly ask "what happens if" in a context carefully specified by the researcher and then record the result.
In Phase 2, we will develop open access calculators and software to calculate our interval, based on formulas and solutions developed in Phase 1. The calculators will downloadable and available online in a website hosted form. We expect that evidence-based practitioners and researchers will benefit from our interval and calculators. Having a clear method to account for measurement error when interpreting research findings will benefit most consumers of research.